Martin Gossar is a lecturer at the Institute of Automotive Engineering, focusing primarily on electrical engineering tasks. One of his particular passions is explaining complex circuits. In this science story, he explores autonomous driving.
Science Story: Autonomous Driving
DI Dr. Martin Gossar, 08. May 2024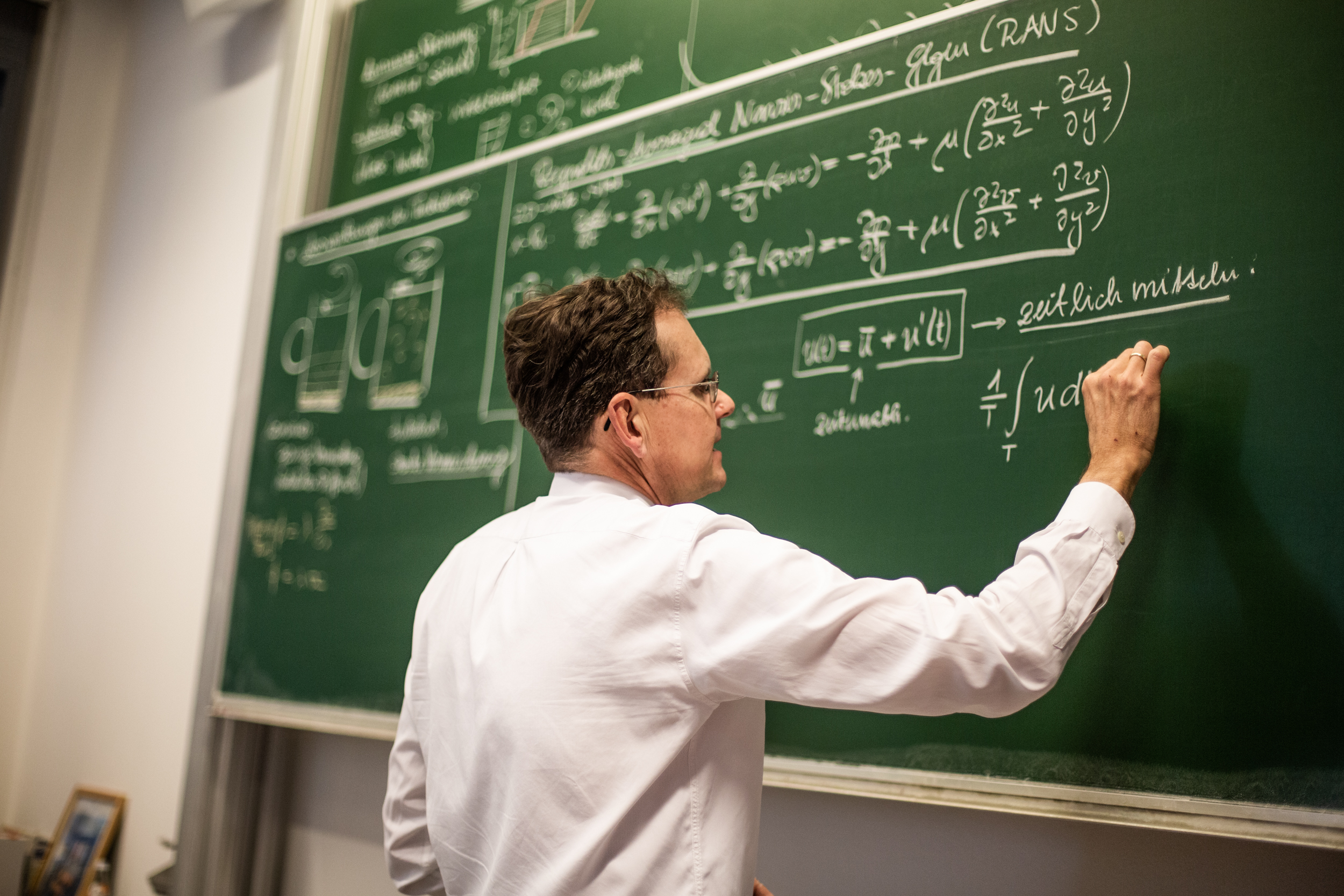
Autonomous driving is a hot topic. For a long time, experts have been grappling with how to make cars move from one point to another autonomously—without human steering. To achieve this, a variety of different tasks must be solved.
📹 Sensing the Environment
First, the vehicle must detect its surroundings and determine where it is allowed to drive and where it is not. This is mostly done today using one or more cameras and various sensors attached to the vehicle. One of these sensors could be an ultrasonic sensor.
The ultrasonic sensor emits very high tones and listens for the echo. We humans cannot hear these tones. By measuring the time between sending and receiving these signals, the sensor can calculate the distance to an object, whether it’s a tree, another car, a person, or a wall. Bats use the same principle to navigate in the dark.
Radar sensors work similarly, but they emit an electromagnetic wave instead of a sound. Lidar sensors emit light pulses but operate on the same principle. Each sensor has specific advantages and disadvantages. Ideally, different sensors are used together to leverage strengths and compensate for weaknesses.
⚠️ Detecting Dangers
Once the environment is captured with cameras and sensors, the vehicle must be able to recognize other road users and assess whether they pose a threat or how to react to them. This is mainly done today through the use of “machine learning.” This involves taking a large number of images and annotating them. This means a person assigns different areas and objects in the image. For example, all cars in the image are marked as cars, all people as people, all trees, signs, houses, etc., as what they are. The more precisely and the more distinctions made, the better an algorithm can subsequently be created to recognize these objects. A multitude of annotated images are presented to the algorithm, which learns to understand its surroundings through this. After a training and learning phase, a neural network is created, which works similarly to our human brain. The more images and the more details on the images that are marked for learning, the better the algorithm will function afterward.
This learning does not happen on individual computers but is outsourced to computer farms. This makes learning much faster but also consumes large amounts of energy.
Since one does not know how effectively the learning has worked, the algorithm is additionally tested with further images. This is to check how safely the algorithm or neural network has recognized different things.
🚗 The Ideal Path
Once the vehicle is able to recognize things and roads, it calculates an ideal path. We do the same when we drive ourselves. We perceive our surroundings and know where we need to drive or how we react when dangers are next to the road.
To implement this ideal path, the car must be able to follow it. A motor steers the wheels, as we would with a steering wheel. When we steer on different surfaces, we feel different resistances. On rough asphalt, there is more resistance than on ice. Or on the highway at higher speeds, we steer differently than when parking. The vehicle must learn this through complex control algorithms. Typically, a multi-stage control is necessary to steer in the desired direction without steering too quickly or jerkily.
We are currently addressing these issues at FH JOANNEUM in the Institute of Automotive Engineering. Through small model cars, we can test recognizing the environment and steering without risk. We have also implemented electric steering in an electric car.